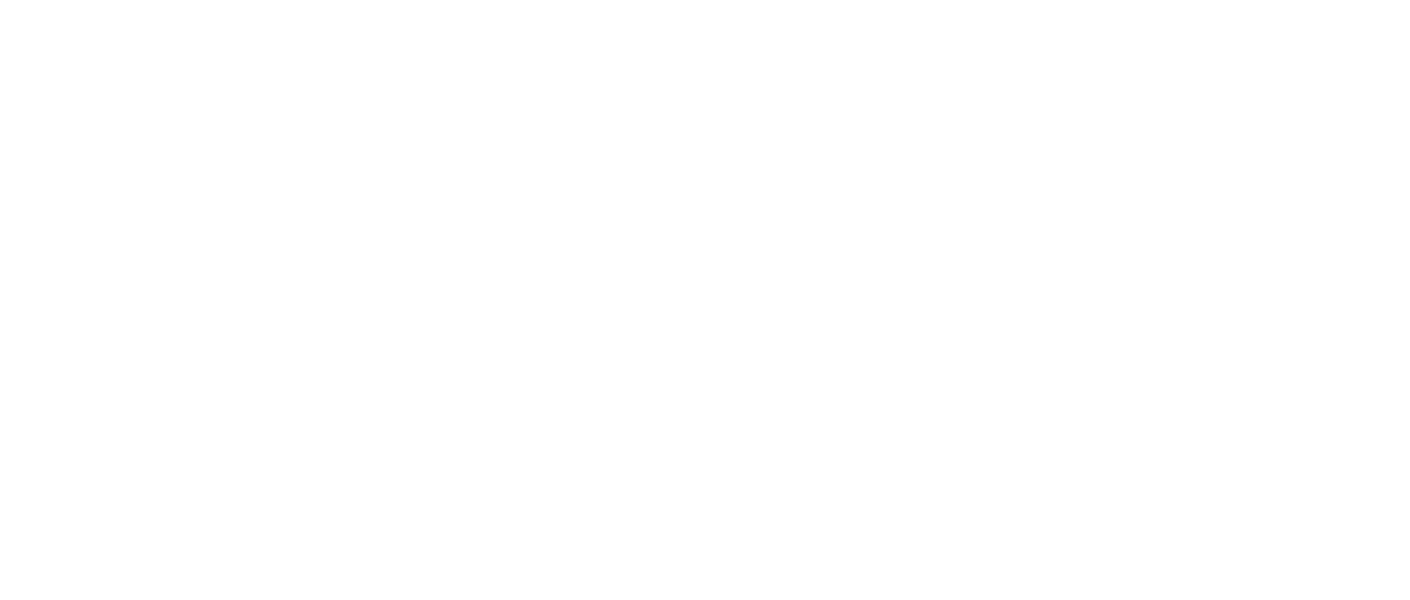
MAETEL, leader of the R+D+i project consortium, DOCTOR-PV
The DOCTOR-PV project will develop a predictive maintenance method with drones in photovoltaic plants, using the most promising technologies.
The DOCTOR-PV project (Development of Optimised Operation and Maintenance Tools for Photovoltaic Plants) is being carried out by a research consortium led by MAETEL, in which Gas Natural Fenosa Engineering, Iberdrola Renovables, PARIVER, Visiona, the Public University of Navarra, the University of Valladolid and CIRCE also participate.
The project is an initiative that aims to improve the performance of photovoltaic plants by means of a new predictive maintenance method, which will take advantage of and combine the most promising techniques on the market in a pioneering manner, allowing early detection of anomalies and identification of faults in photovoltaic plants in a fully automated manner. The combination of these methodologies will allow better planning of operation and maintenance work, as well as anticipation of failures, reducing energy losses associated with malfunctions and optimizing their overall performance.
This project, with file number RTC-2017-6712-3, has been financed by the Ministry of Science, Innovation and Universities - State Agency and co-financed by the European Union through the European Regional Development Fund (ERDF), with 1.1 million Euros, with an execution period of two and a half years (July 2018 - December 2021), within the call RETOS-COLABORACION 2017 that supports projects in cooperation between companies and research organizations, whose objective is to promote technological development, innovation and quality research.
The project combines the most promising techniques related to predictive maintenance, maintenance based on the condition of all plant elements and electroluminescence (EL) and infrared thermography (IRT) measurements, both combined and implemented in drones to perform inspection tasks at the same time in the same flight, being one of the great innovations of this R+D+i project.
In order to carry out predictive maintenance based on the condition, "machine learning" methods will be used that allow early detection of faults in the main components of the plant.